New paper on using machine learning to model oxygen and hydrogen isotopes in precipitation published in PNAS
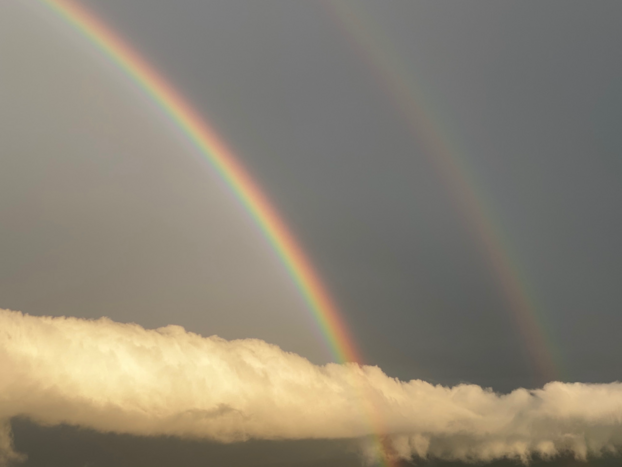
Dan Nelson led the study with contributions from David Basler and Ansgar Kahmen, which used a new modelling approach based on machine learning to predict monthly oxygen and hydrogen isotope values in precipitation beginning in 1950. Stable isotopes in precipitation can be used as a chemical tracer in many diverse applications in biological and environmental sciences. This includes, for example, understanding when and where plants access their water supplies, validating agricultural food product origin using isotope forensics, and improving interpretations of paleoclimate data from tree rings, cave deposits, or lake sediments. The model presented in this study provides more accurate reconstructions of precipitation isotope values than have previously been available, and makes these available for any location in Europe through a simple web-based interface (link below).